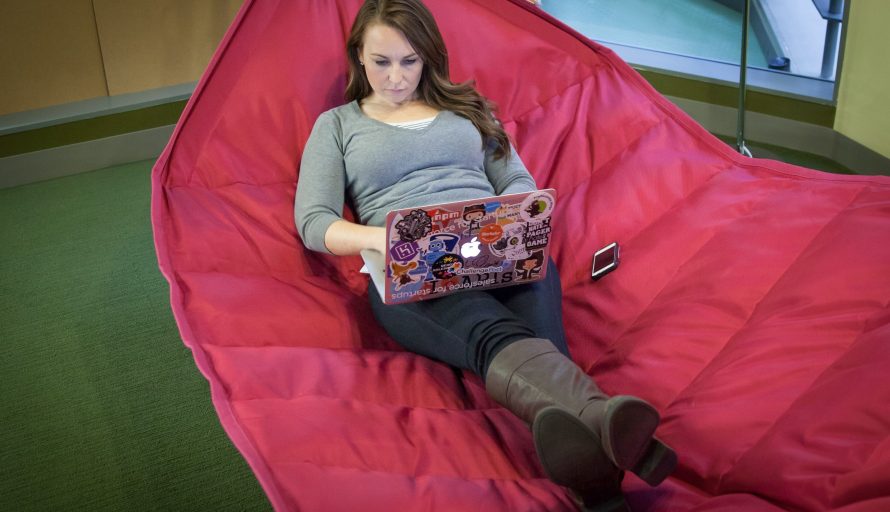
Today in email marketing, the time that an email is sent has a high impact on user engagement. Sending at an optimal time can help drive more successful and effective campaigns. In order to send at the best time, you need to have a good understand of your users’ email engagement pattern. Sending right before the time a user interacts with their inbox can lead to the highest chance of engagement.
We analyzed user email open and click behaviors across thousands of our participating customers, billions of users, and hundreds of billions of email events across a three month window in 2021 and found out that the engagement pattern not only varies by brand and geolocation, but also, most importantly, from individual to individual.
Having a system that constantly monitors user behaviors, adaptively adjusts the strategy according to user feedback, and reasonably balances between exploration and exploitation can truly help lift email engagement over time. This is the general rationale behind our Einstein Send Time Optimization feature. Here’s a deep dive into the analysis we did to build the algorithm that drives the feature for our customers.
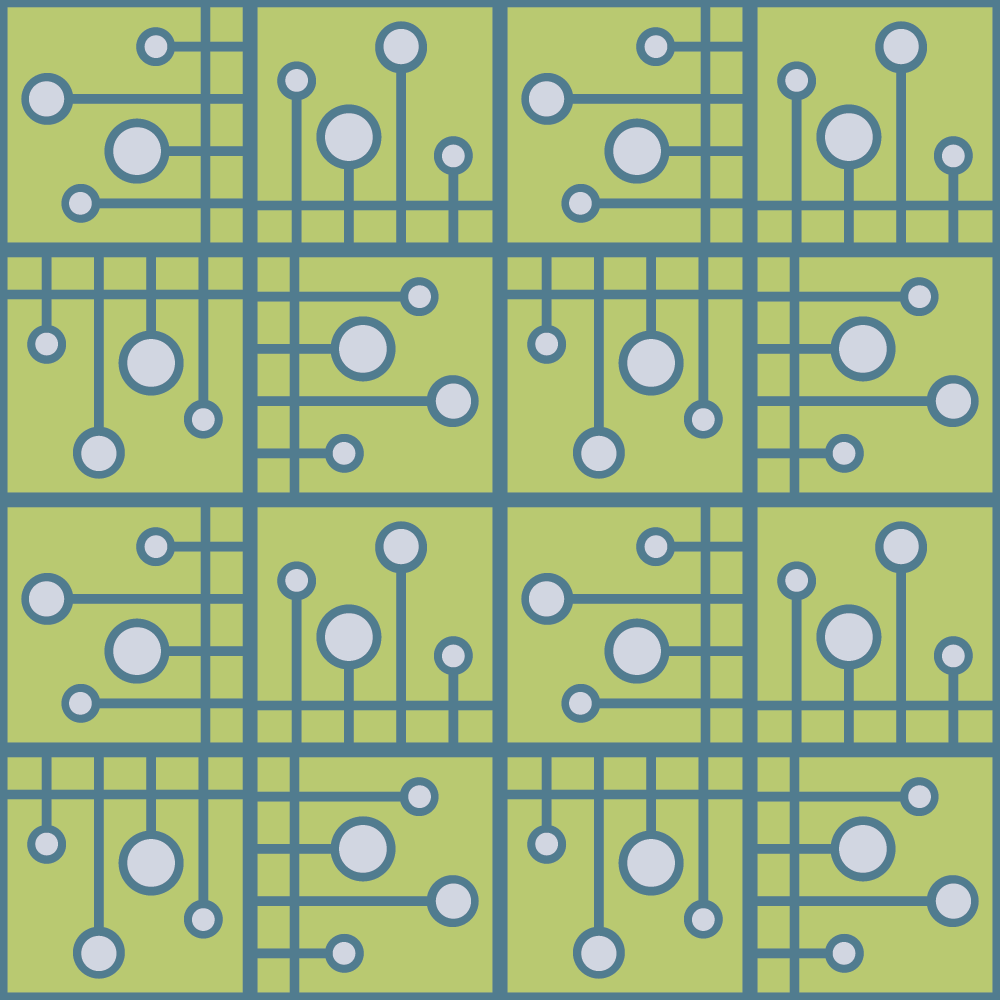
When do people open their emails?
First of all, let’s look at when users open their emails throughout the week.
The chart below shows an aggregated view of hourly email opens for the entire week across all participating customers. With respect to Central time (CT), mornings (especially 8am ~ 10am) consistently have higher number of opens compared to other times of the day, and weekdays tend to have more opens than weekends. This is a good start to understand general behavior; however, is that enough? Each of our customers generally have audiences with different behaviors. In order to answer that question, we need to drill down into the analysis and look at the customer level.

When we drill down to customer level, the email open patterns start to show differences. Some audiences of those customers have more opens over the weekend than weekdays (e.g. Customer B). The spikiness also varies (e.g. opens in Customer C are more spread out than in Customer A and B).
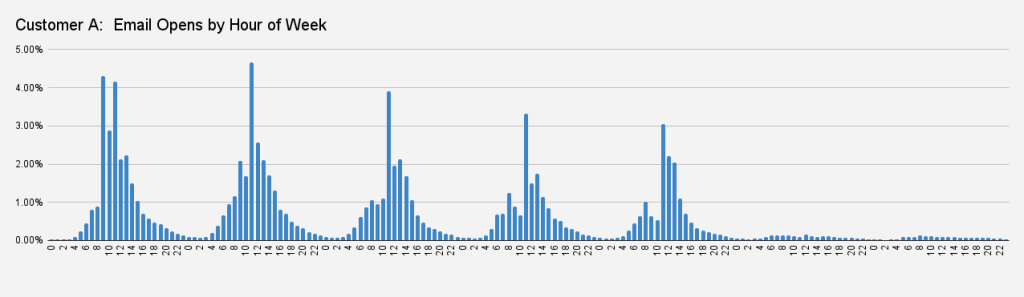

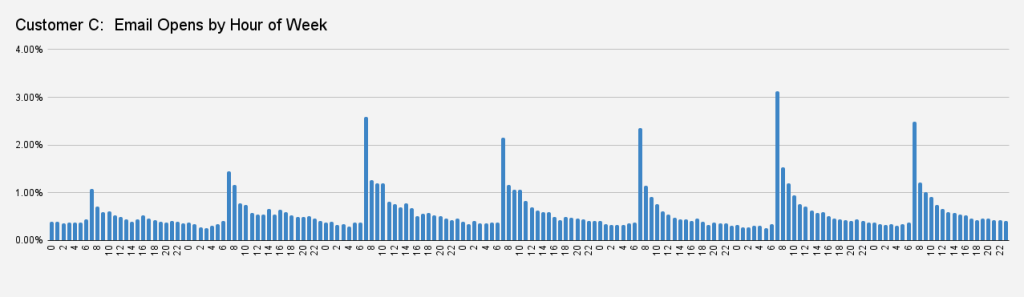
So now that we know the customer’s audience behavior patterns, shall we pick the time with highest opens and send our emails?
That seems reasonable at first glance, and this is what most send time optimization systems on the market do. However, thinking it a bit deeper, it’s not hard to see that such simple aggregation ignores users’ unique individual behavior. Simply sending based on customer level results is not optimal.
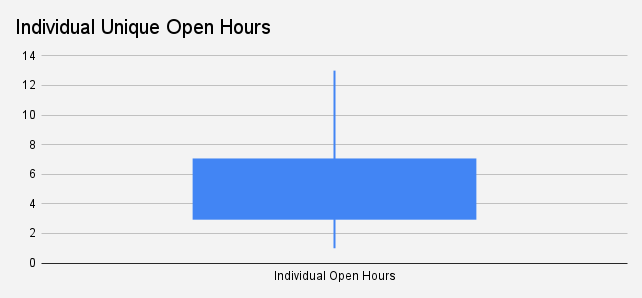
Our research shows that 75% of users only open their emails at no more than seven unique hours throughout the week. Though customer level opens may establish certain patterns, when we drill down to individual behaviors, it becomes quite spotty and unique. One user’s best open time may be another user’s least engaging time, and vice versa. This further demonstrates that having personalized send time is the key to more effective and successful marketing campaigns.
How to generate personalized send time
There are many different factors that influence personalizing the best time to send to your audience. How engaged the users are, the geolocations, devices, email clients, etc. may all play a role. Among all these potential factors, behavioral patterns is the most direct and informative influencing factor.
1. Emphasis on personal behaviors
Personal engagement data is the most powerful metric to know when individuals are most and least engaged with their emails. Having a system that constantly monitors and captures these types of data can help identify both sweet spots and times to avoid when reaching out to your audience. Engagement data here include opens, clicks, unsubscribes, etc., and each may carry different degree of engagement for different types of campaigns or businesses. Customized engagement metrics can be designed to suit different business needs.
2. Utilize customer specific characters
Since the vast majority of users engage at only a few distinct hours, merely relying on individual engagement data is not enough. We need other information to fill in the blanks. One way is to infuse the customer level aggregated open data to make up for the hours that an individual user does not have engagement data for. Make sure that it’s less emphasized in your model than personal behaviors so that personal engaging hours are still the most outstanding. If more granular level data is available, such as business unit or segment level, it can also be utilized at certain importance degree to collaboratively generate personalized send time.
Another advantage of customer level engagement patterns is that it is cold-start friendly. When new users enter into the system that we don’t have any prior knowledge about, customer level engage patterns can be a better guide than sending at random time.
3. Take into account open delays
Up to now we have been mainly focusing on when users open emails. However, sending at individuals’ open time may not always lead to maximum engagement. One important component that can not be neglected is the lag from send time to open time.

The graph captures how delayed email opens are from the time emails reach to users’ inbox. We can see that around 30% opens happen within the first hour, while more than 50% emails have significant open delay.
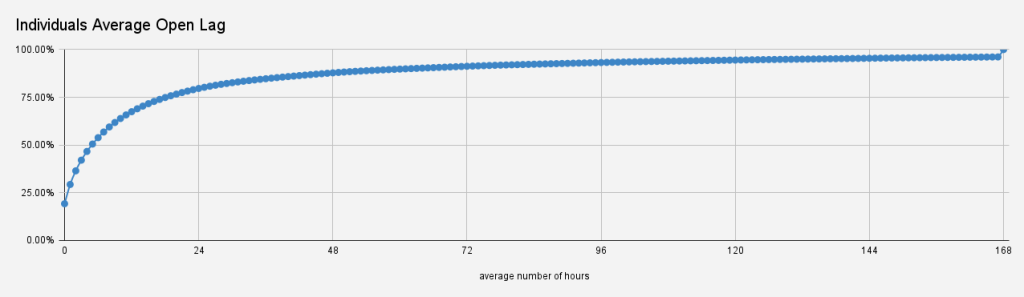
From another perspective, our analysis shows that individual users’ average open lag varies a lot. More than 70% users have significant open delay.
So shall we shift the send time ahead to allow for the average open lag? The answer is: it depends!
For users that consistently show relatively stable open lag, subtracting the delta to derive the optimal send time can provide good results. For those harder-to-predict individuals, we need to be more careful when doing so. Things can be even trickier when individual data is not very large. It’s quite easy to draw biased conclusions based on occasional observations.
To summarize, the best time to send emails is quite individualized and dynamic. Customer level engagement data can help reveal some user engagement patterns at an aggregated level. The most engaging time does not always equal to the best send time. Our system is built with Spark in a distributed fashion to scale for customers with various ranges of data volume. In order to best tailor the unique characteristics of each customer, we use an assembly model structure to provide optimal recommendations for all of our customers. Our detailed analysis into how people engage with their emails, coupled with machine learning, allows us to help our customers service their customers with emails delivered at exactly the right time for them.