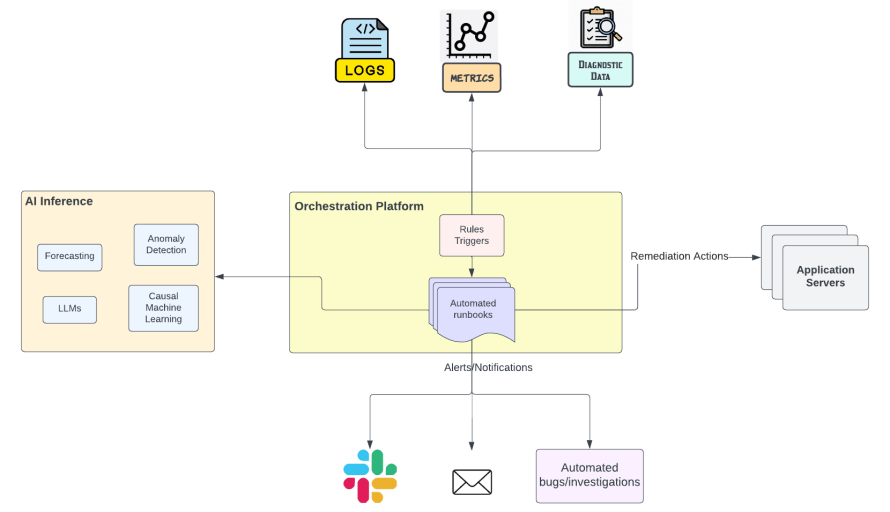
In our “Engineering Energizers” Q&A series, we explore the remarkable journeys of engineering leaders who have made significant contributions in their respective fields. Today, we meet Sravanthi Konduru, a Lead Member of the Technical Staff for Salesforce Engineering, who helps drive the development of the Warden AIOps platform.
Explore how her team overcame challenges to incorporate cutting-edge automation and AI technologies — significantly streamlining the workload of human analysts while ensuring the business continuity across Salesforce production environments.
How would you describe the Warden AIOps platform?
Our AIOps platform integrates automation and AI, serving as an intelligent assistant that helps Salesforce teams streamline monitoring and management of production environments. By collecting and analyzing real-time data such as metrics, logs, diagnostic reports, and events from all applications, it proactively identifies and mitigates potential issues before they impact customers. This significantly reduces downtime and minimizes the need for human intervention.
With our platform, the process of collecting and analyzing observability data, detecting incidents, and performing remediation is simplified. It offers a customizable, self-service, plug and play framework for internal customer teams to tailor the AIOps system to their specific requirements. This empowers teams with limited programming experience to easily write code and define rules for if specific issues arise.
Sravanthi explains her role on the AIOps platform team.
What role does automation and AI play in your AIOps platform?
Automation eliminates the need for human intervention by handling predefined tasks and running workflows through automated runbooks. It manages about 30% of incidents by monitoring various data sources and performing incident remediation. This relieves internal teams from dealing with known and repeated incidents related to their service.
For incidents beyond automation’s capabilities, the platform’s AI-based causation engine takes input from automation’s initial diagnosis, correlates it with past incident data, and recommends mitigation strategies. The AI solves an additional 30% of issues using human-like analysis. The remaining 40% of incidents are edge cases where AI suggests potential causes and mitigation to human analysts for review and decision-making.
Combining automation and AI significantly reduces the workload on human operators, saving thousands of manual hours per year. This allows the platform to handle new environments without requiring additional human resources, even as Salesforce’s production environment grows.
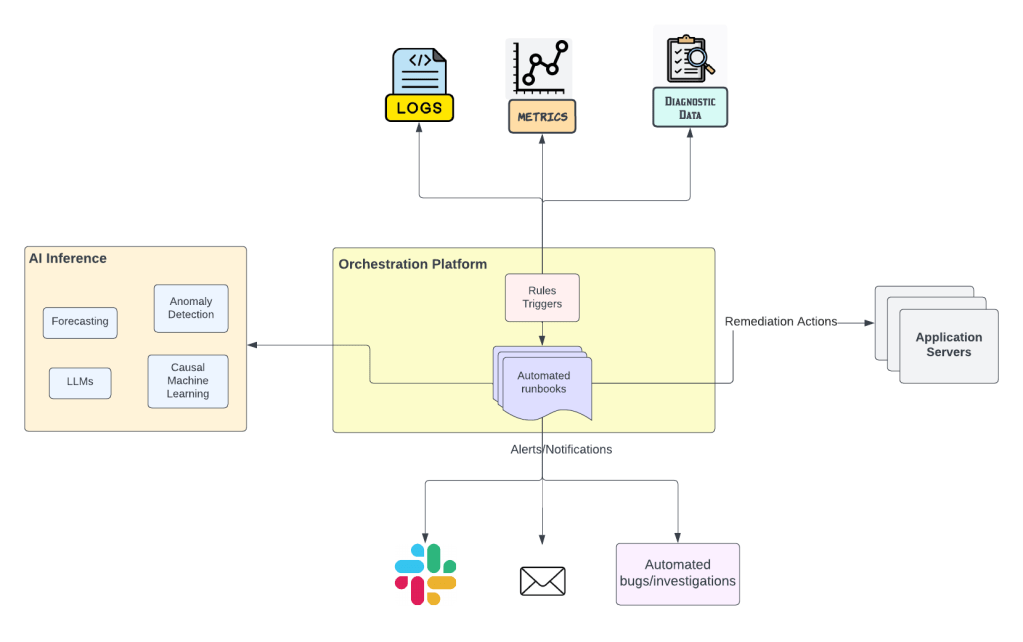
A high-level look at the AIOps platform architecture.
What was the biggest AI challenge your AIOps team faced?
Improving the coverage and accuracy of our AI models in incident triaging, recommending, and mitigation posed a significant challenge. To address this, we focused on continuous training and updating of our models to ensure their relevance and accuracy over time.
One notable incident we encountered involved our AI model’s inability to recommend solutions for symptomatic incidents like average page time (APT) degradation. We discovered that the AI lacked sufficient inputs from our predefined runbooks and had insufficient information about these incident types.
To overcome this, we took three steps:
- We enhanced the automated runbooks, which gave initial diagnosis to the AI model.
- We provided the AI model with more incident data, enabling it to learn from a wider range of examples.
- We ensured the data provided to the model was clean and up-to-date, as outdated or inaccurate data could hinder the model’s performance.
By retraining the model with improved data and enhancing the automated runbooks, the AI was able to better identify and understand these specific incidents, resulting in more accurate recommendations.
Sravanthi shares what keeps her at Salesforce Engineering.
What automation challenges did your team encounter?
Ensuring the security of auto remediation actions in a production environment was one of our major challenges. To overcome this obstacle, we collaborated closely with our security team, obtaining security approvals for training AI models, determining data inputs, and ensuring that the blast radius of auto-remediation is minimum to avoid wide spread impact if anything goes wrong.
However, obtaining security approvals within Salesforce is a rigorous process due to the high priority placed on trust. Initially, the security team had concerns about our proposed design and architecture, prompting them to suggest alternative, more secure approaches.
We iterated and worked closely with them to navigate the approval process, ultimately delivering a robust solution that met safety and security standards while ensuring an excellent customer experience.
What prompted the development of your AIOps platform?
It was largely prompted by the limitations of traditional operations, where site reliability engineers had to manually monitor dashboards for issues within Salesforce production environments.
As Salesforce grew, the manual effort required to manage these environments became unsustainable. This led Salesforce to rethink its approach to operations. Applying automation and AI to the data became a natural extension, enabling faster, more efficient analysis and providing valuable recommendations for problem resolution.
How does your team continuously improve the efficiency and accuracy of your AIOps platform?
We employ several tactics:
- Add runbooks: To increase efficiency, we constantly strive to incorporate more runbooks and make the AI smarter. By onboarding more runbooks, we can improve detection and automate more scenarios.
- Discover opportunities for improvement: Our monthly reporting system is designed to uncover any potential coverage gaps in our AIOps. By identifying incidents that may have been missed, we can proactively collaborate with our service teams to gain a deeper understanding of why these incidents occurred. This process enables us to continuously enhance our operations and ensure comprehensive coverage.
- Retraining and validation: We collaborate with the Salesforce AI Research Team to improve the accuracy of AI-based remediation recommendations. While they continuously retrain the AI models and validate their recommendations with human input, we provide them with domain knowledge, data, and requirements for the models. This iterative process ensures that the AI models are tailored to our specific needs and expertise.
Sravanthi explores Salesforce Engineering’s culture.
How does your AIOps team measure the efficiency of your AIOps platform?
Our team evaluates the efficiency, effectiveness, and impact of our AIOps platform using standard metrics such as MTTD (Mean Time To Detect), MTTT (Mean Time To Triage) and MTTR (Mean Time To Remediate). The goal is to improve MTT metrics every year by leveraging AIOps. We also measure the number of incidents prevented by AIOps through prompt automated actions.
Additionally, we automatically generate bug reports for code owners based on post-remediation analysis of diagnostic data, enabling prompt resolution and prevention of future incidents.
What’s next for your AIOps platform?
We are currently working on enhancing our AIOps platform with the introduction of an AIOps Copilot framework. This framework will be equipped with conversational capabilities, allowing operational teams to easily inquire about ongoing incidents using the Copilot bot. The goal is to streamline incident resolution by providing a platform that can dynamically generate queries to fetch monitoring data, autonomously analyze the data, and take remediation actions. Our aim is to empower operational teams with expedited incident resolution and a range of other functionalities.
Learn More
- Stay connected — join our Talent Community!
- Check out our Technology and Product teams to learn how you can get involved.